Generative Artificial Intelligence (AI) stands at the forefront of technological innovation, pushing the boundaries of what machines can achieve. Unlike traditional AI systems that operate based on predefined rules, generative AI possesses the ability to create and generate new content, often indistinguishable from human-produced work. In this article, we will unravel the intricate workings of generative AI, exploring how it transforms data into creative outputs and revolutionizes various industries.
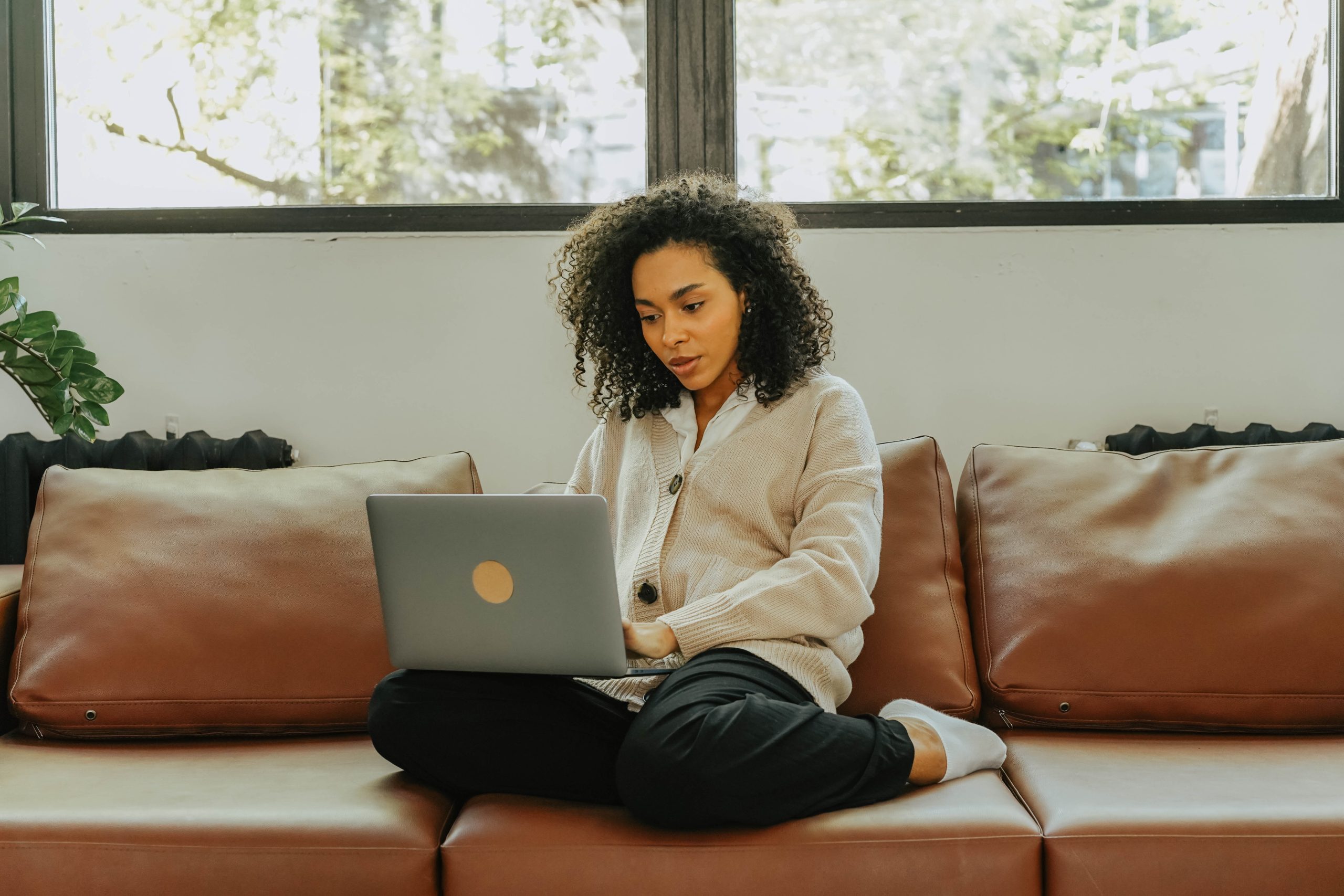
1. Understanding Generative AI
a. Definition
– Generative AI refers to a class of algorithms and models that have the capacity to generate new, original content autonomously. This content can span a wide array of forms, including text, images, music, and even entire virtual environments.
b. Key Distinction
– The fundamental distinction between generative AI and other AI systems lies in its ability to produce outputs without being explicitly programmed for a specific task. Instead, it learns patterns and structures from existing data.
2. Neural Networks and Generative Models
a. Neural Networks
– At the core of generative AI are neural networks, computational models inspired by the human brain. These networks consist of interconnected nodes, or neurons, that process and analyze data.
b. Generative Models
– Generative models, a type of neural network, learn the underlying patterns and features of a dataset. This enables them to generate new instances that share characteristics with the original data.
3. Types of Generative Models
a. Variational Autoencoders (VAEs)
– VAEs focus on learning a probabilistic representation of the input data. They can generate new data points by sampling from this learned distribution.
b. Generative Adversarial Networks (GANs)
– GANs consist of two neural networks – a generator and a discriminator – engaged in a competitive process. The generator creates content, and the discriminator evaluates its authenticity. This adversarial training refines the generator’s capabilities over time.
4. Natural Language Processing (NLP) and Text Generation
a. Transformers in NLP
– For text generation, transformer models have emerged as powerful tools. These models, such as OpenAI’s GPT (Generative Pre-trained Transformer), learn to predict the next word in a sequence, enabling them to generate coherent and contextually relevant text.
b. Contextual Understanding
– Generative AI in NLP goes beyond simple word prediction; it understands the context of the entire input sequence, allowing for the creation of human-like text that adheres to grammar, style, and context.
5. Image Generation and Style Transfer
a. Conditional GANs
– In image generation, Conditional GANs are widely used. These models can generate images based on specific conditions, such as a textual description or a set of input parameters.
b. Style Transfer
– Generative AI can also perform style transfer, transforming the artistic style of an image while retaining its content. This is achieved by separating and recombining content and style representations.
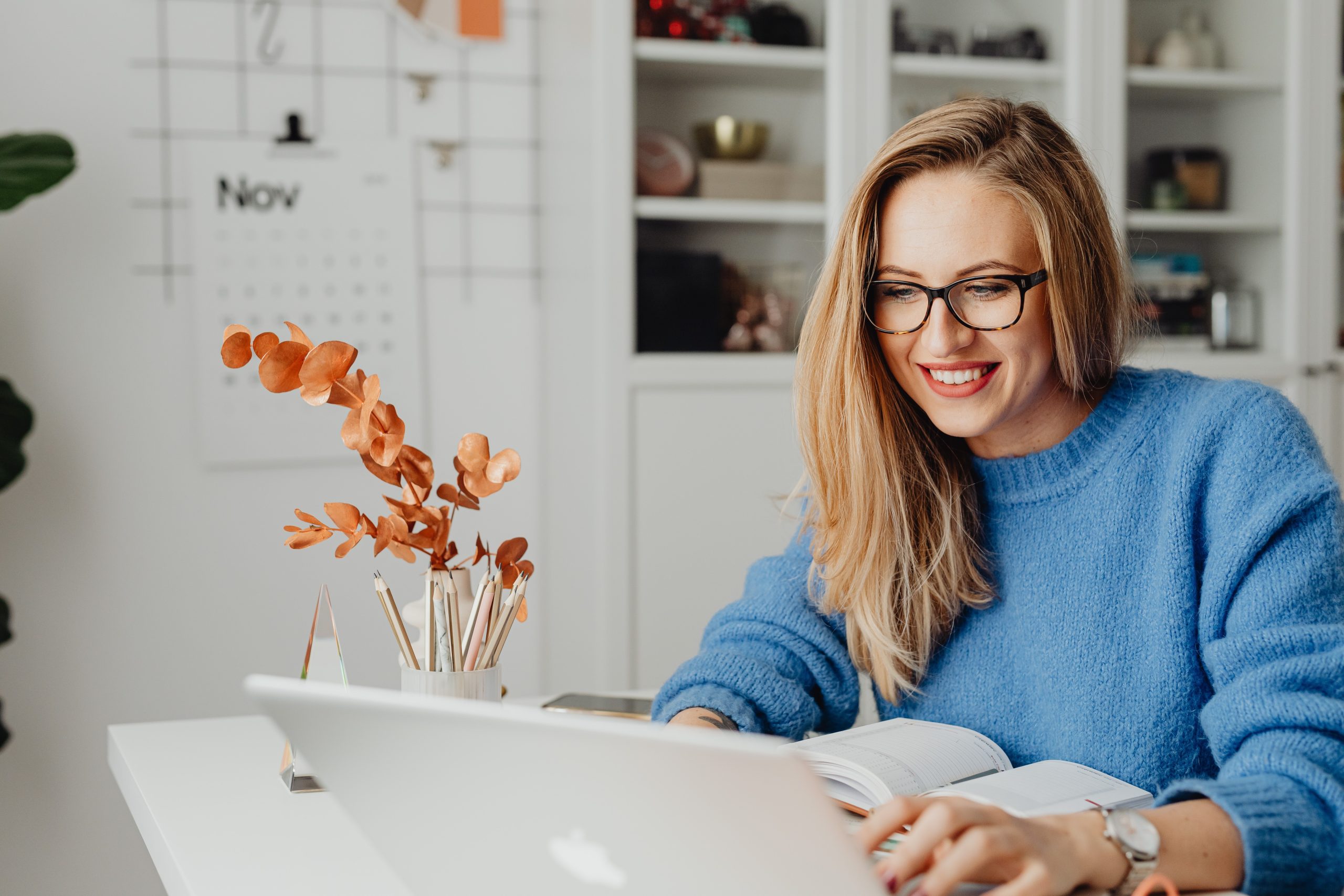
6. Applications of Generative AI
a. Art and Creativity
– Generative AI has found a home in the world of art, creating paintings, music, and poetry that captivate and challenge traditional notions of creativity.
b. Content Creation
– In content creation, generative models assist in generating text, images, and videos, streamlining workflows for marketers, writers, and designers.
c. Virtual Environments
– In virtual environments, generative AI contributes to the creation of realistic scenes and characters, enhancing the gaming and simulation industries.
7. Challenges and Ethical Considerations
a. Bias in Data
– Generative AI models are susceptible to biases present in the training data, which can result in the generation of biased or discriminatory outputs.
b. Deepfakes and Misinformation
– The rise of deepfakes, powered by generative AI, poses challenges related to the potential misuse of this technology for creating deceptive content and spreading misinformation.
8. The Future of Generative AI
a. Continual Advancements
– As research in generative AI progresses, continual advancements are expected, leading to more sophisticated models capable of understanding and generating increasingly complex and nuanced content.
b. Human-AI Collaboration
– The future of generative AI envisions a collaborative relationship between humans and machines, where AI augments human creativity and aids in solving complex problems.
Generative AI stands as a testament to the remarkable capabilities of modern artificial intelligence. By leveraging neural networks and generative models, this technology has transcended traditional programming boundaries, unlocking a realm of creative possibilities. From transforming the way we generate text and images to reshaping entire virtual environments, generative AI is shaping the future of industries across the spectrum. However, as we embrace the potential of generative AI, it is crucial to address ethical considerations and ensure responsible development and deployment. The journey of generative AI is still unfolding, promising a future where machines and creativity intertwine in ways previously unimaginable.