As artificial intelligence continues to revolutionize natural language processing (NLP), the quest for transparency and interpretability in model decision-making has never been more vital. Enter xai770k, an advanced explainable AI (XAI) model specifically designed to address this gap in complex NLP tasks. With its blend of cutting-edge technology and interpretability layers, xai770k is reshaping the way we understand how machines comprehend and generate human language.
Unlike traditional black-box language models, which often produce results with limited insight into their internal reasoning, xai770k is built with explainability at its core. This gives researchers, developers, and users a clearer window into how specific outputs are derived, underlining trust and accountability in NLP-powered applications.
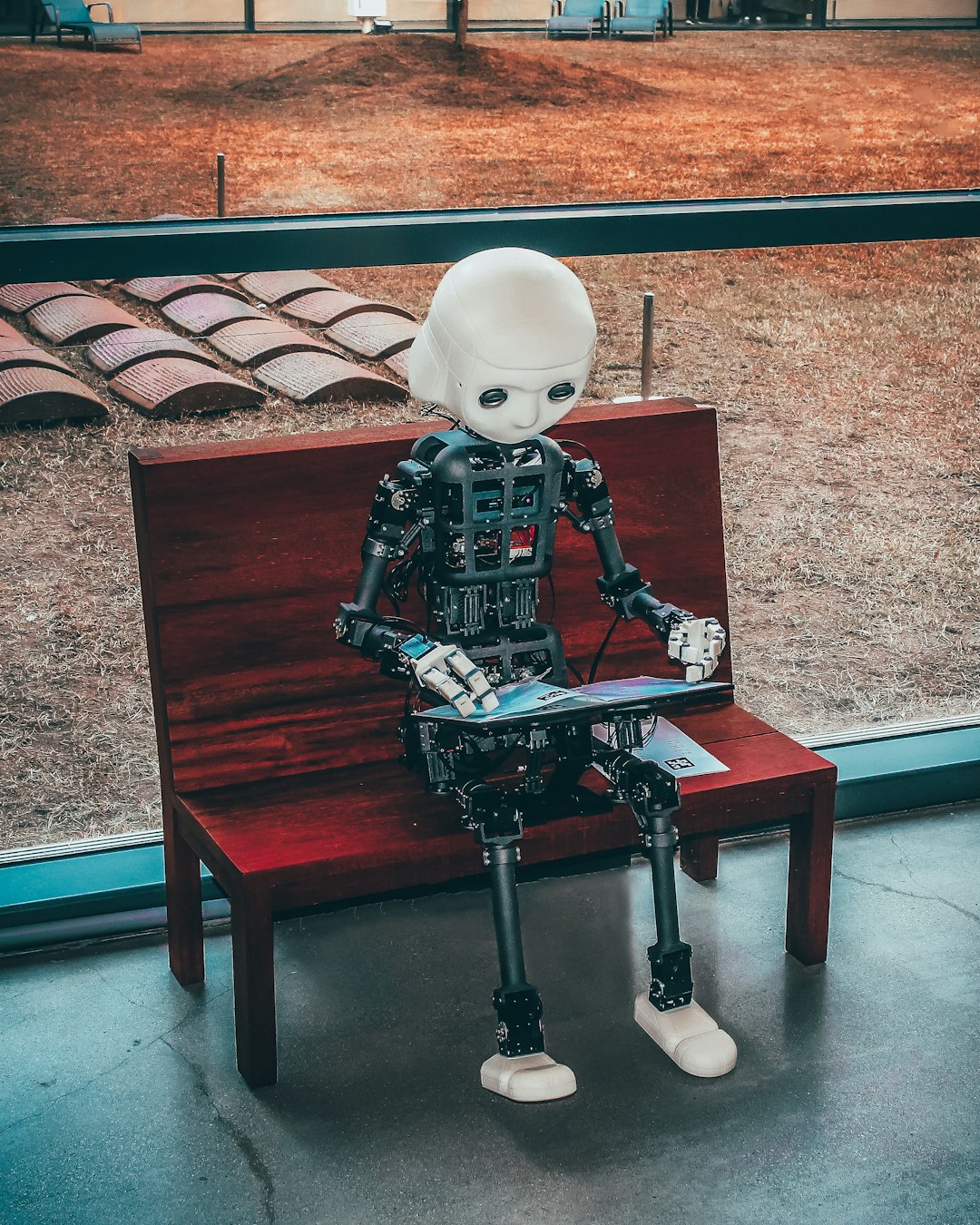
The Framework Behind xai770k
The architecture of xai770k is built upon a hybrid transformer-based model enriched with interpretability mechanisms. These include:
- Attention Heatmaps – Visual representation of words or phrases that the model focused on during processing.
- Token Attribution – Methods like Integrated Gradients and SHAP that assign relative importance to input elements.
- Semantic Reasoning Modules – Layers designed to deconstruct the input into logical components for better traceability.
These elements work together to make xai770k not just a high-performing model but a highly understandable one, ideal for scenarios where decisions must be justified — such as healthcare, legal tech, and finance.
Real-World Applications of xai770k
Explainable NLP models are crucial in sensitive domains where AI-generated decisions need human-level scrutiny. xai770k stands out in these areas:
- Legal Document Analysis – Offers transparency in clause identification and precedent mapping.
- Medical NLP – Enables interpretability in diagnostic suggestions based on patient histories.
- Financial Forecasting – Clarifies language-based indicators influencing prediction models.
In a court setting, for instance, lawyers and analysts depend on AI suggestion tools to identify critical statutes and past judgments. xai770k’s exhaustive breakdown offers a clear lineage of how a conclusion was reached — from word emphasis to contextual interpretation.
How It Outperforms Traditional Models
While powerful black-box models like GPT and BERT have dominated the NLP space recently, they often fall short in delivering why a certain response or classification is made. xai770k bridges this gap by maintaining strong performance without sacrificing explainability. Key advantages include:
- Fine-Grained Interpretability – Breaks down sentence-level reasoning better than generic attention-based models.
- Adaptive Transparency – Adjusts the level of detail in its explanations based on the complexity of the NLP task.
- Integration Flexibility – Easily embedded into existing NLP pipelines with optional explanation toggles.
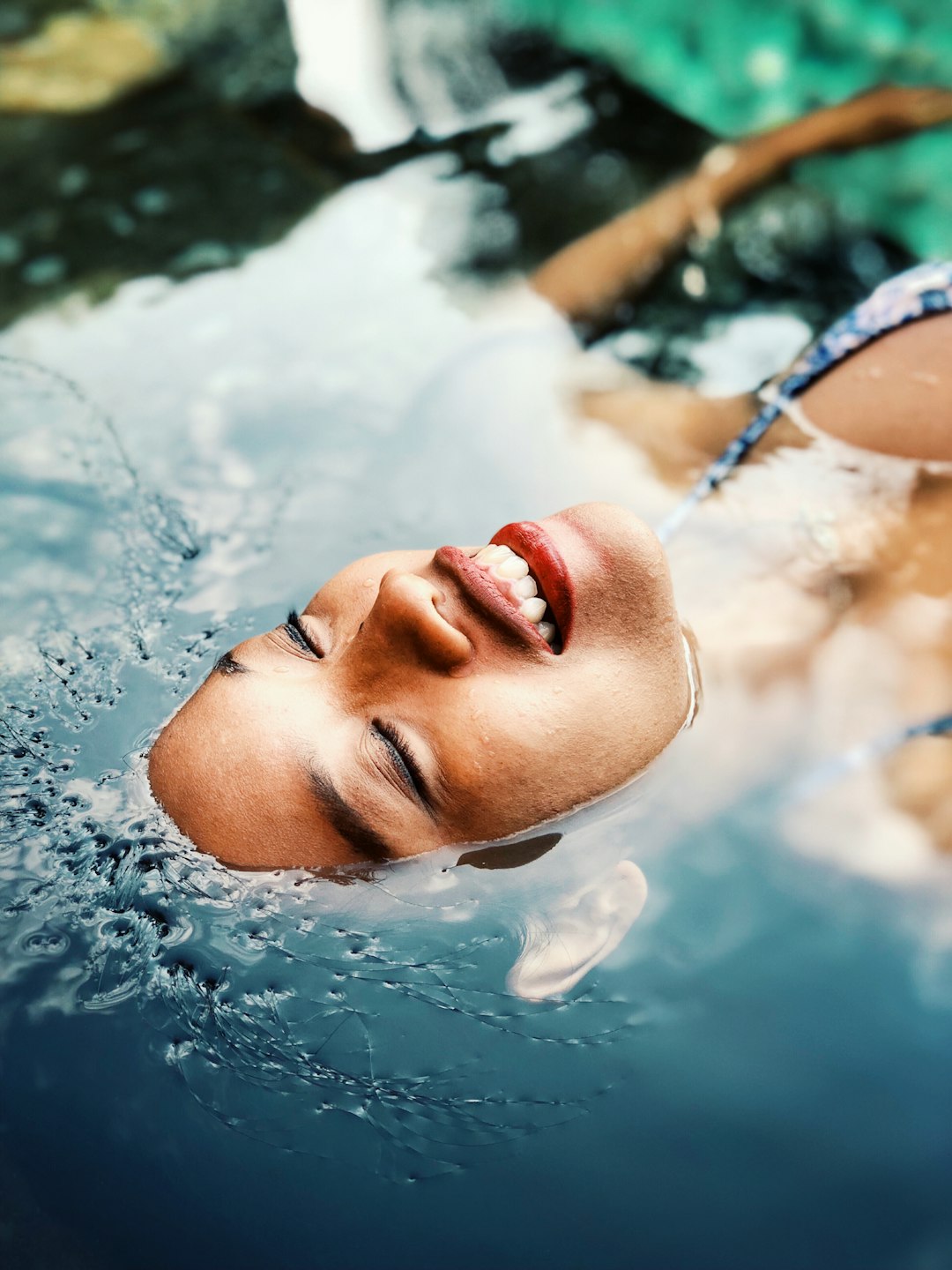
These capabilities make xai770k a preferred model for teams addressing both technical and ethical AI concerns. By illuminating linguistic nuance within data, the model supports better product development, user trust, and compliance with emerging AI regulations.
Challenges and Considerations
Despite its many strengths, building and deploying explainable models like xai770k comes with its own set of difficulties:
- Performance Trade-Offs – Adding interpretability components may impact speed compared to more opaque models.
- Explanation Overload – Not all users need or understand deep explanatory output, which can complicate UI design.
- Data Dependency – The quality of explanations is directly tied to training data bias and coverage.
Nevertheless, with proper tuning and context-aware deployment strategies, these challenges can be mitigated, allowing xai770k to deliver meaningful, actionable insights across a diverse set of tasks.
The Future of Explainable NLP with xai770k
As regulatory pressure mounts for “responsible AI,” models like xai770k are leading the charge toward more ethical and accountable language technologies. Future iterations are expected to introduce multilingual explainability layers, real-time feedback loops from users, and even more granular semantic trace mechanisms.
With its innovative design and practical utility, xai770k is not just a model — it’s a movement toward a future where AI doesn’t just talk back, but also explains itself clearly and trustworthily.